Unlocking the Potential of Labeling Tools for Machine Learning
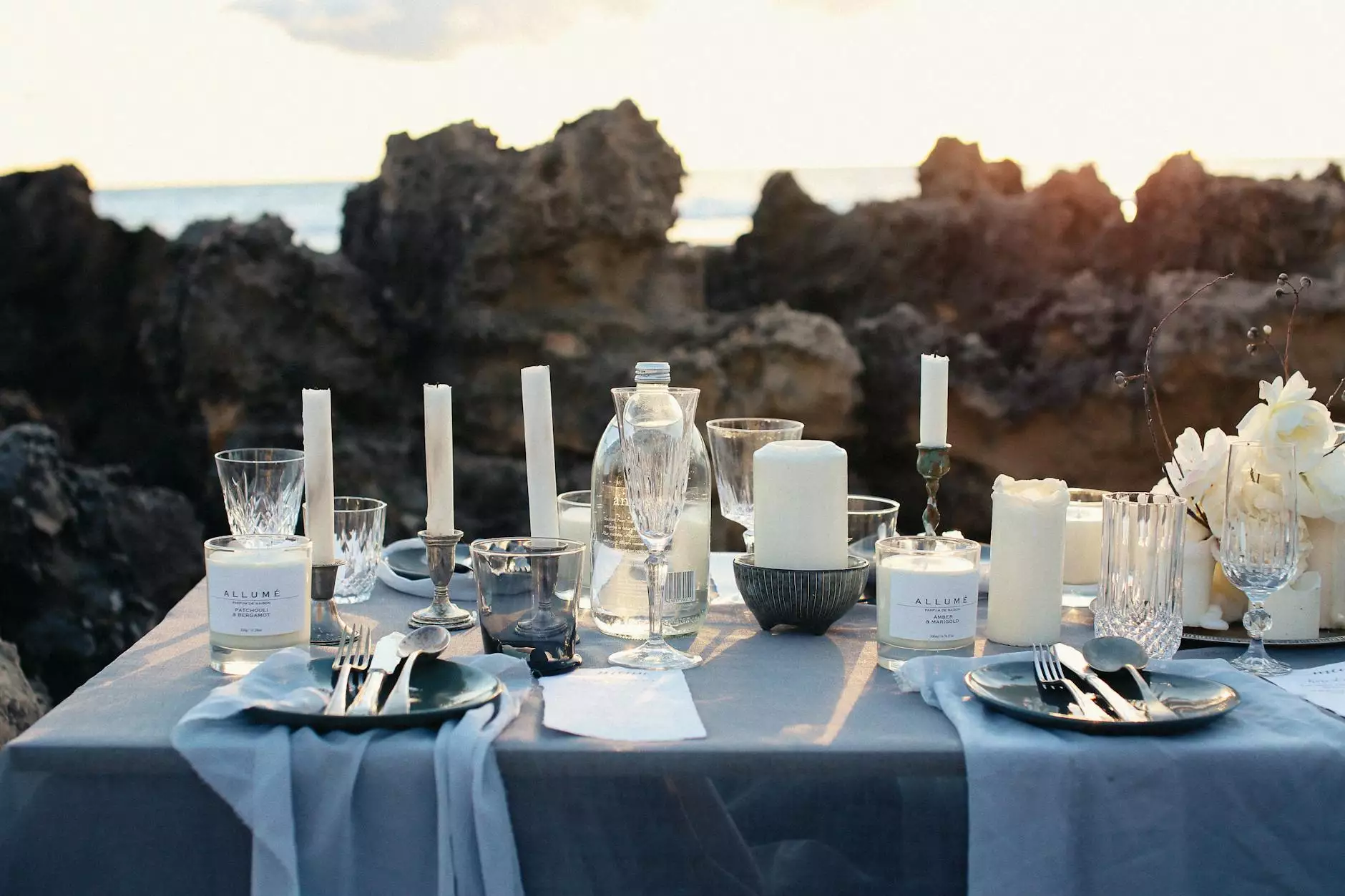
In today's rapidly evolving technological landscape, the incorporation of machine learning into various industries has revolutionized how businesses operate. In particular, the field of home services, including keys and locksmiths, has seen significant transformations due to the application of intelligent algorithms powered by machine learning. At the heart of this evolution lies the need for effective data labeling, achieved through labeling tools for machine learning.
The Importance of Data Labeling in Machine Learning
Data labeling is a critical process in the field of machine learning, where raw data is annotated to provide context and meaning. This process ensures that algorithms can learn from recognizable patterns in the data, which is crucial for tasks such as image recognition, natural language processing, and predictive analytics.
- Enhanced Accuracy: Well-labeled data leads to higher accuracy in model predictions.
- Training Efficiency: Efficient labeling processes speed up the training time of machine learning models.
- Scalability: Automated labeling tools allow businesses to scale their operations rapidly.
Understanding Labeling Tools for Machine Learning
When it comes to labeling tools for machine learning, several options cater to different needs. These tools enable businesses to tag and organize data efficiently, ensuring that machine learning models are trained with high-quality inputs. Here are some popular types of labeling tools:
1. Image Annotation Tools
For businesses in home services, such as keymakr.com, image annotation tools are invaluable. They allow users to add labels to images, which can be used in applications like object detection or image classification.
- Examples:
- Labelbox
- SuperAnnotate
- VGG Image Annotator
2. Video Annotation Tools
In scenarios where video data is prevalent, annotating videos for events, objects, or actions is essential. This can be especially useful for training algorithms in surveillance systems for locksmith security.
- Examples:
- CVAT (Computer Vision Annotation Tool)
- Vatic
3. Text Annotation Tools
Text data requires specific labeling tools to categorize and annotate written content. These tools can transform unstructured text into actionable insights, especially beneficial for customer feedback analysis in home services.
- Examples:
- Amazon SageMaker Ground Truth
- Prodigy
Benefits of Using Labeling Tools for Machine Learning
Integrating labeling tools into your machine learning pipeline can provide numerous benefits, particularly for home service businesses like keymakr.com.
1. Consistency and Quality
Automated and semi-automated labeling tools ensure a high level of consistency and quality in the data being labeled, which is crucial for the performance of machine learning models.
2. Cost-Effectiveness
By reducing manual labor and speeding up the labeling process, these tools can significantly lower costs associated with data preparation.
3. Faster Time-to-Market
With quick and efficient data labeling, businesses can bring machine learning-powered solutions to market faster, gaining a competitive edge.
Implementing Labeling Tools in Your Business Strategy
For businesses in home services such as locksmithing, incorporating labeling tools into your operational strategy can be transformative. Here’s how you can embark on this journey:
1. Assess Your Data Needs
Evaluate the types of data you currently have and the specific labeling requirements that arise from your machine learning projects.
2. Choose the Right Tool
Select a labeling tool that aligns with your specific needs, budget constraints, and technical capabilities.
3. Train Your Team
Ensure that your team understands how to effectively use the chosen tool, which may include training sessions and workshops.
Best Practices for Data Labeling
To maximize the effectiveness of labeling tools for machine learning, adhere to the following best practices:
- Establish Clear Guidelines: Create a systematic approach to data labeling to maintain consistency.
- Quality Assurance: Implement a review process to ensure the accuracy of labeled data.
- Leverage Automation: Utilize machine learning-assisted labeling features to accelerate the process without compromising quality.
Case Studies: Successful Implementation of Labeling Tools
Numerous companies have successfully integrated labeling tools to improve their machine learning operations. Here are a few notable examples:
1. A Home Security Company
This company leveraged video annotation tools to enhance their surveillance algorithms. By efficiently labeling security footage, they improved threat detection accuracy, positively impacting their service delivery.
2. A Property Management Service
By using image labeling tools, this service could categorize properties based on features such as size, location, and amenities. This data-driven approach allowed for better marketing strategies and improved customer satisfaction.
The Future of Labeling Tools for Machine Learning
The future of labeling tools is promising, with advancements in artificial intelligence, automation, and cloud technologies continuously shaping the landscape. As machine learning models grow more sophisticated, the need for high-quality labeled data will only intensify.
1. Increased Automation
Expect to see a rise in fully automated labeling tools that can pre-label data based on learned patterns, reducing the need for manual input.
2. Integration with AI
Tools that integrate seamlessly with AI systems will enhance the labeling process, providing intelligent insights as data is being labeled.
Conclusion
In summary, labeling tools for machine learning are indispensable for businesses looking to harness the power of data in today’s market. For home service companies like keymakr.com, these tools not only enhance operational efficiency but also elevate service quality. By implementing effective labeling strategies, businesses can unlock new opportunities and maintain a competitive edge in a data-driven world.
As the demand for machine learning applications increases, investing in the right labeling tools should be a top priority for companies aiming to succeed in the digital age.